オフィスワークで使用する文具について思うこと
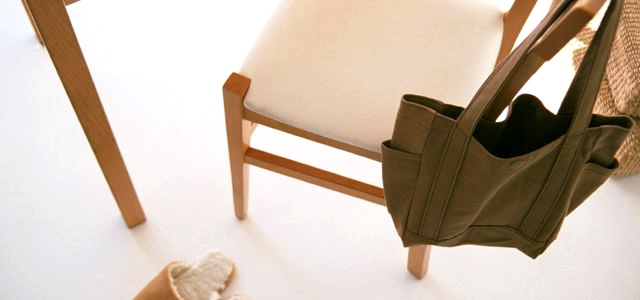
仕事にも慣れてきた最近、思うのは、毎日仕事に使う文具についてです。当たり前ですが、会社で使用する文具というのは、オーソドックスな落ち着いたデザインが一般的です。事務員の女性だけではなく、男性社員が使っても、なんら違和感のないデザインのものではあります。オフィスワークになって半年、毎日見る文具たちに、遊び心が出始めていたのでした。休日の楽しみはショッピングで、お洋服だけではなく、文具を見るのも趣味の一つです。真面目な普通の文具ではなく、女子向けの雑貨屋さんで販売されているような、キュートでコミカルさもある、あの可愛い文具たちです。毎日会社で見るクリップとは異なり、雑貨屋さんで見るクリップには、アニマル型もあります。ペンギンの形、犬や猫の形と、こんな文具だったら、仕事ももっと楽しくなるな、と考えるのです。クリップひとつとっても、会社の黒いゴツイ大型クリップも、雑貨やで見るものは、ピンクやパステルカラーの、スイーツ型など、思わずほっこりしてしまいます。せめて自分のデスクで使うものだけでも、遊び心のある文具を使いたいとひらめきました。
まずは使いきりのミニ付箋を、アニマルデザインのものを選んで会社で使ってみました。会社の経費で文具は購入しますが、自分のモチベーションアップのため、自分で購入して使っています。これが結構ウケて、付箋のメモより、カワイイ付箋に喜ぶ女子社員は多かったです。いずれ役職が付いたら、文具についても提案したいと考えています。